Dr. Chintan Amrit
See profileMayukh Ghosh
See profileEstimating Child Wasting Burden in the Sahel Region using Ensemble Machine Learning Methods
Reliable and timely estimates of global acute malnutrition are required for policy decisions and planning for the provision of treatment and prevention services
Estimating Child Wasting Burden in the Sahel Region using Ensemble Machine Learning Methods. A collaboration between Analytics for a Better World, World Food Programme and Northeastern University.
Wasting, or acute malnutrition increases a child’s risk of infection and death and if suffered repeatedly or for long periods, reduces their ability to learn, be productive and live a healthy life. Reliable and timely estimates of global acute malnutrition (GAM, combining all forms and severity levels of acute malnutrition) are required for policy decisions and planning for the provision of treatment and prevention services. In the absence of incidence data, the GAM burden is estimated as a function of prevalence and an, often standard, incidence correction factor. However, obtaining a reliable estimate is challenging as the incidence varies throughout the year and by geographic location, thereby often leading to an underestimation of the burden.
In our study, in collaboration with the World Food Programme (WFP) and researchers from Northeastern University’s department of Mechanical and Industrial Engineering, we propose novel multivariate ensemble classification models to predict the burden of GAM in the Sahel region of Africa to strengthen ongoing regional efforts to improve lean-season planning and response. These methods were well received by WFP, and results should now be presented to regional partners for validation and discuss how these methods could be incorporated into the regional planning tools.
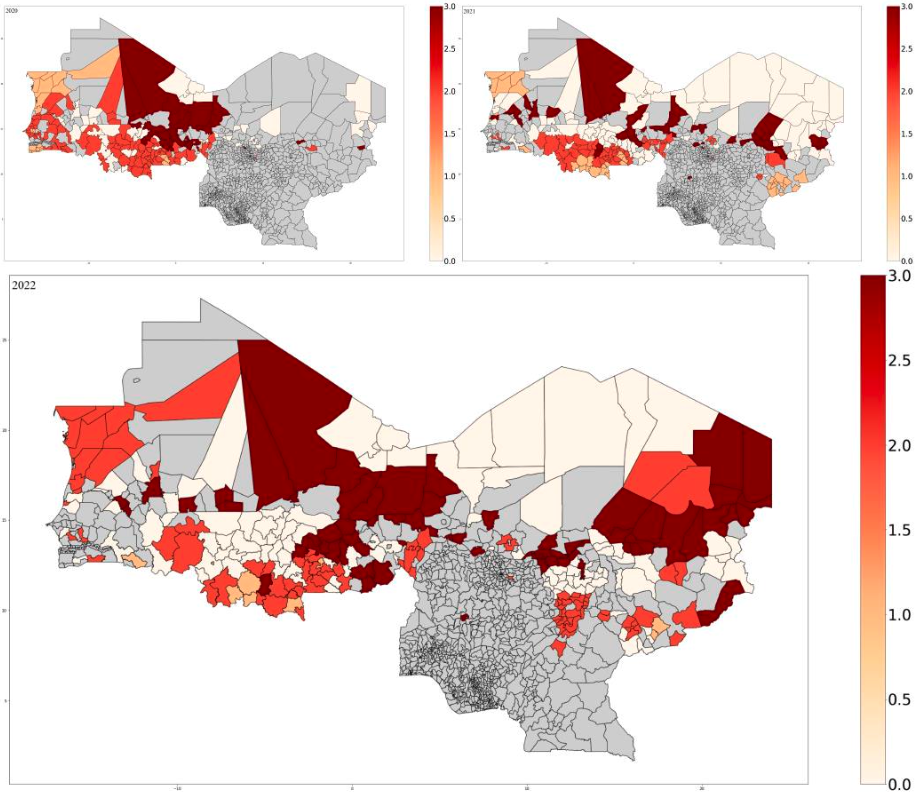
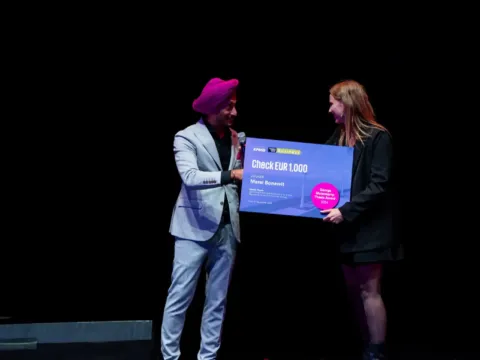
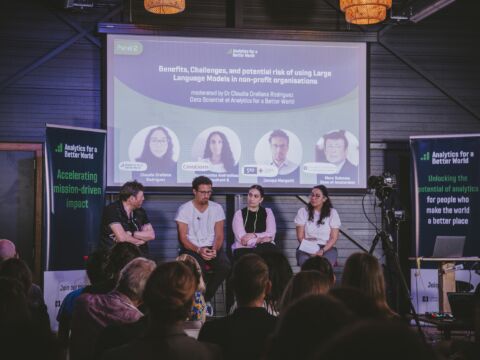
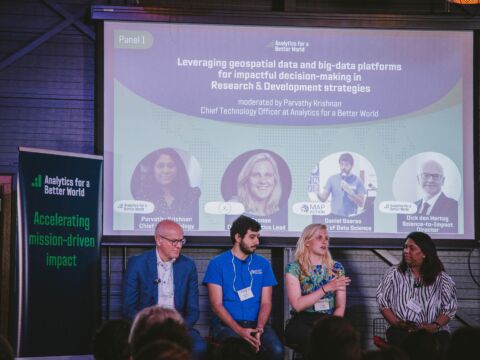
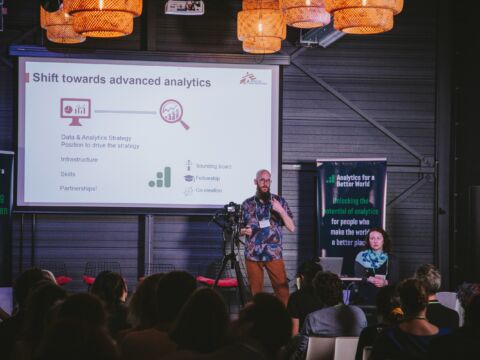
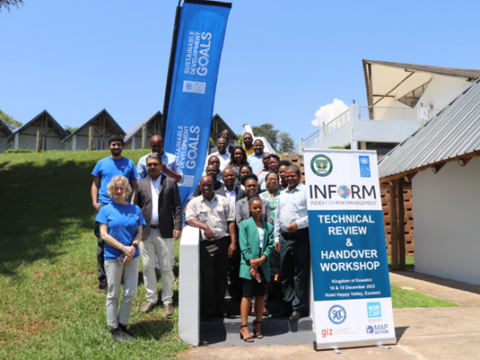
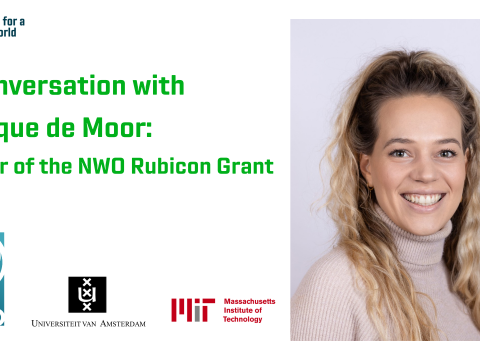
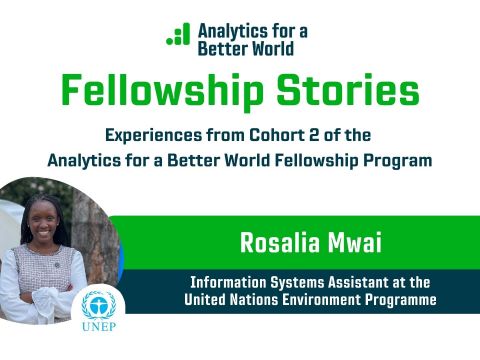
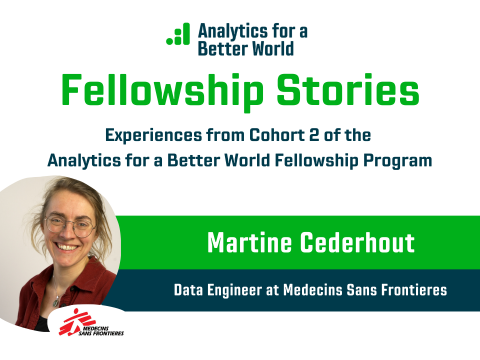